Building Your First Convolutional Neural Network With Keras
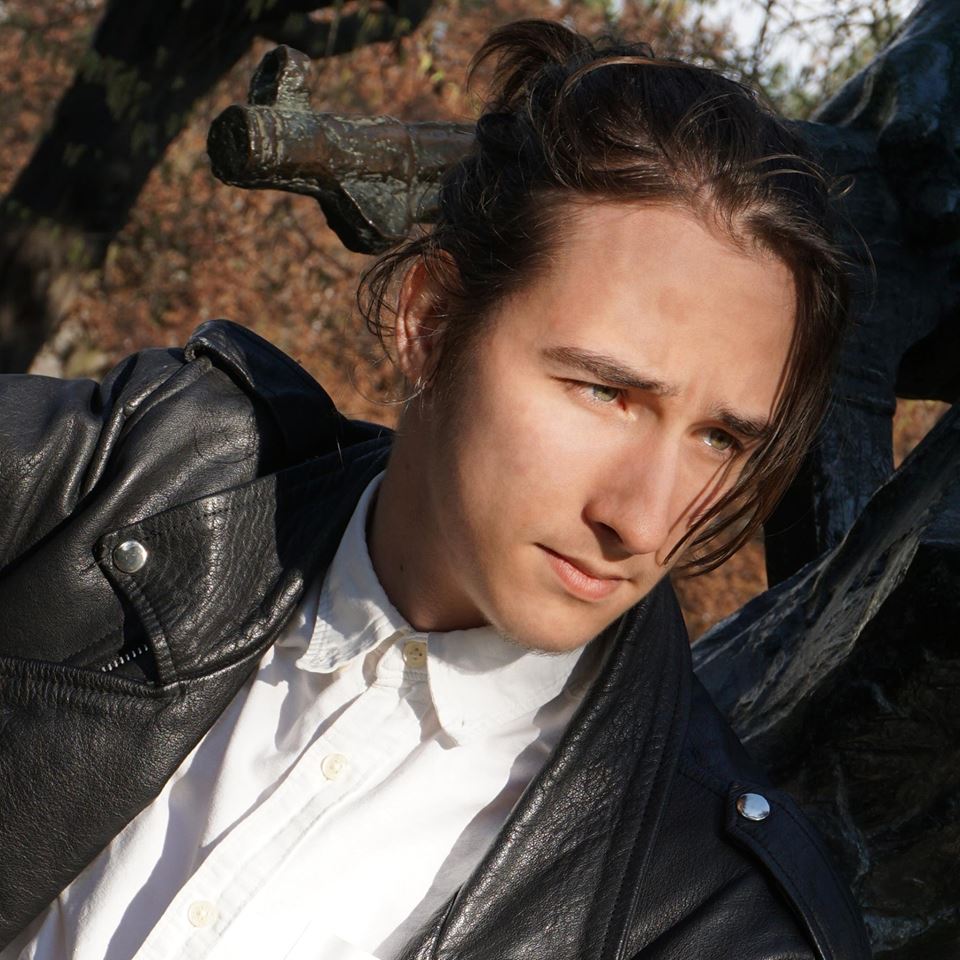
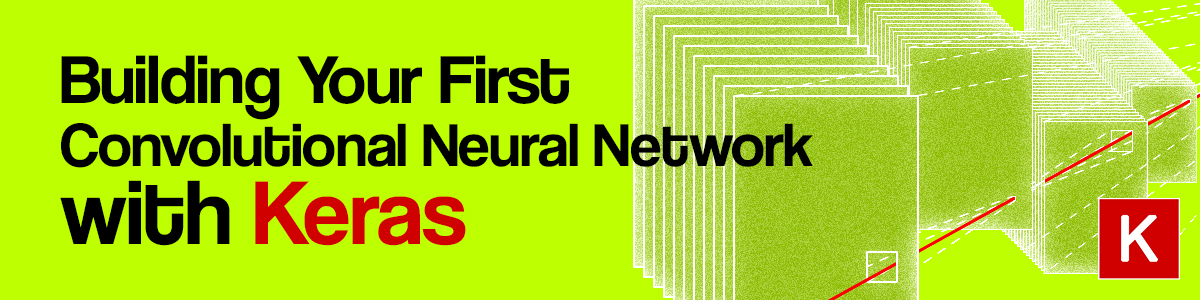
Overview
You may know how to build a CNN - but do you understand it?
Most resources start with pristine datasets, start at importing and finish at validation. There's much more to know.
Why was a class predicted? Where was the model wrong? What made it predict wrong? What was the model's attention on? What do the learned features look like? Can we separate them into distinct classes? How close are the features that make Class N to the feature that make Class M?
Here's the latent feature space of your model visualized, and otherwise hidden away from you:
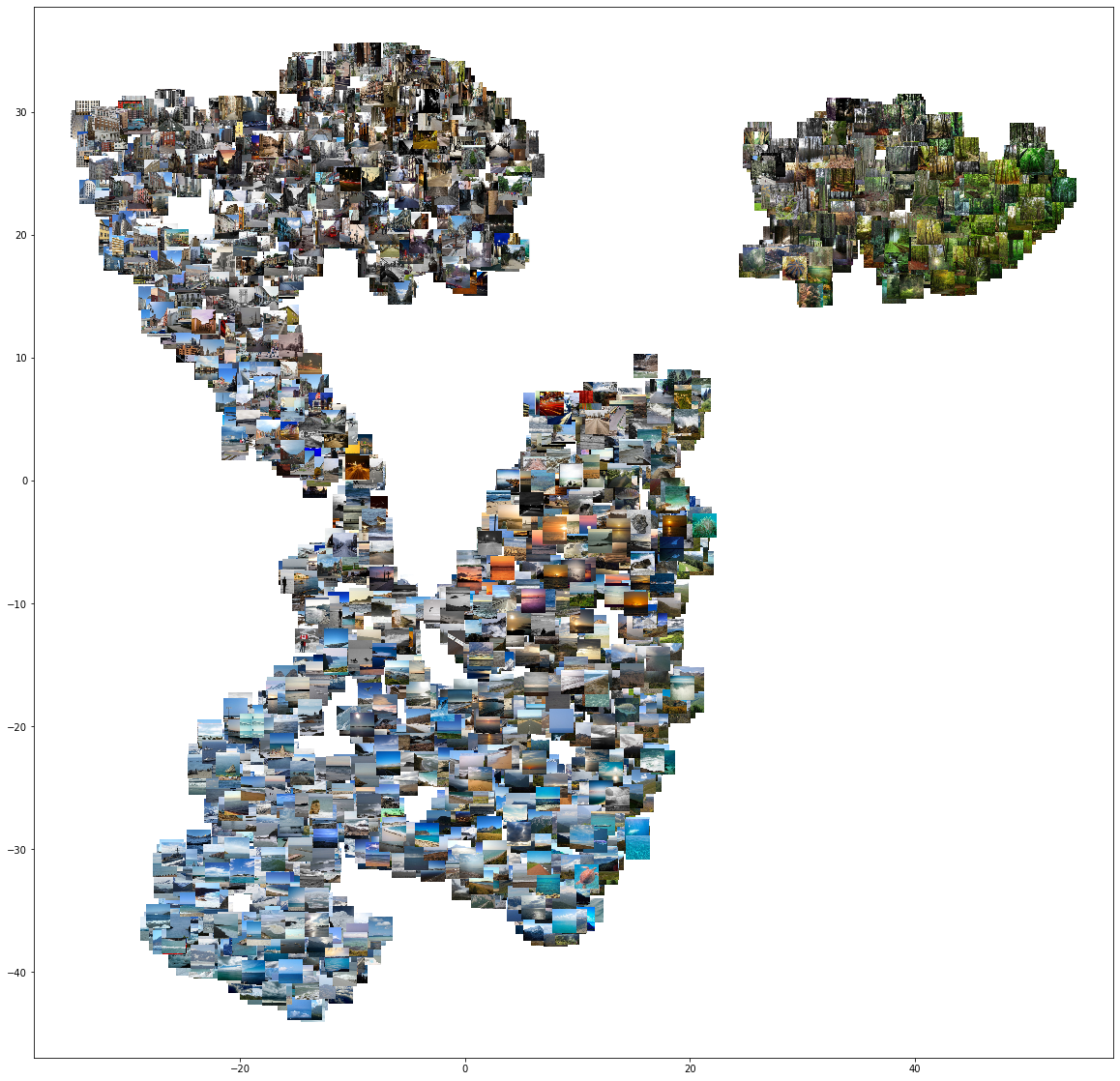
You're new to Deep Learning for Computer Vision and have gone through the theory and basics or you feel unsure about how to build Convolutional Neural Networks?
Literature is vast, and either it's too long and theoretical or too brief to be practical. In this Guided Project - we'll go through the process of building your own CNN using Keras, assuming you're familiar with the fundamentals.
In this project, through a practical, hand-held approach, you'll learn about:
- Co-occurrence and the source of co-occurrence bias in datasets
- Finding, downloading datasets, and extracting data
- Visualizing subsets of images
- Data loading and preprocessing
- Promises and perils of Data Augmentation and Keras' ImageDataGenerator class
- Defining a custom CNN architecture
- Implementing LRFinder with Keras and finding learning rates automatically
- Evaluating a model's classification abilities
- Interpreting a model's predictions and evaluating errors
- What makes the network predict wrong
- Interpreting a model's attention maps to identify what models actually learn with tf-keras-vis and GradCam++
- Interpreting what the model's convolutional layers have learned through Principal Component Analysis and t-SNE
- How similarity search engines find similar images
Note: This Guided Project is part of our in-depth course on Practical Deep Learning for Computer Vision and assumes that you've read the previous lessons or have that prerequisite knowledge from before.
Lessons
Downloadable Resources
Project Jupyter Notebook(10 MB) | Enroll to download |
What is a Guided Project?
Turn Theory Into Practice
All great learning resources, books and courses teach you the holistic basics, or even intermediate concepts, and advise you to practice after that. As soon as you boot up your own project - the environment suddenly isn't as pristine as in the courses and books! Things go wrong, and it's oftentimes hard to pinpoint even why they do go wrong.
StackAbuse Guided Projects are there to bridge the gap between theory and actual work. We'll respect your knowledge and intelligence, and assume you know the theory. Time to put it into practice.
When applicable, Guided Projects come with downloadable, reusable scripts that you can refer back to whenever required in your new day-to-day work.