Practical Deep Learning for Computer Vision with Python
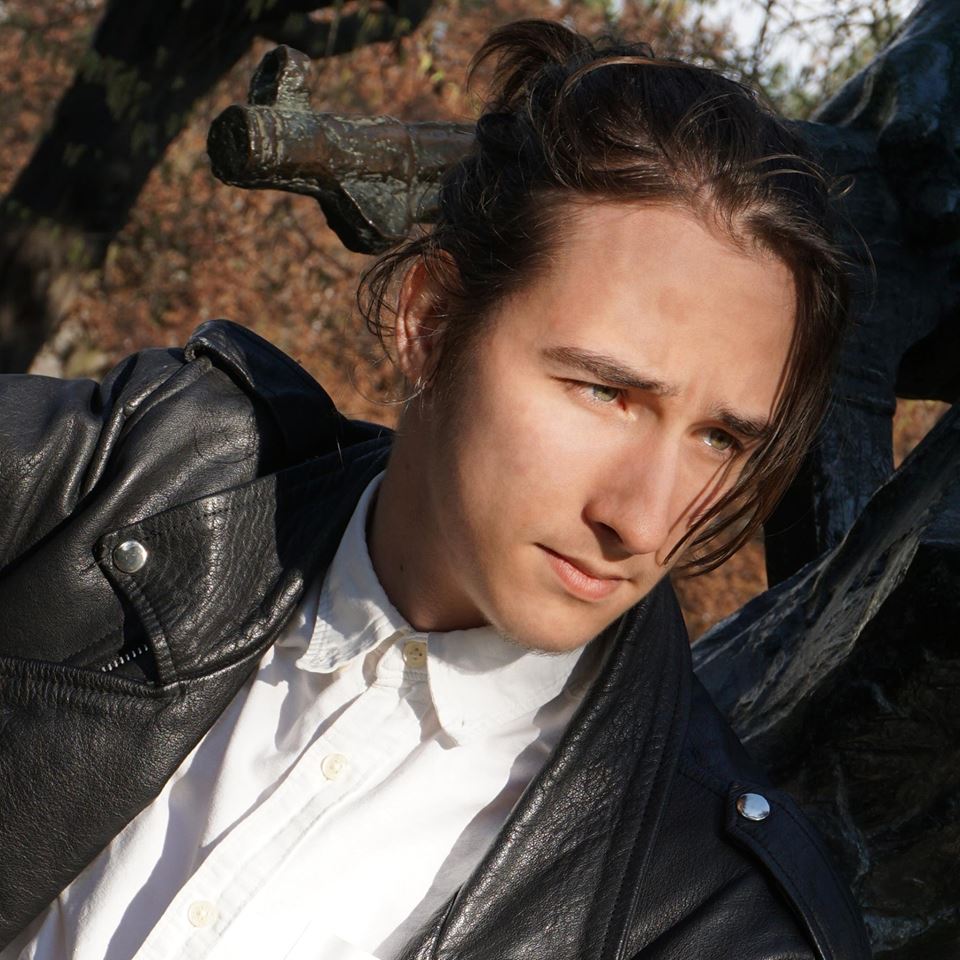
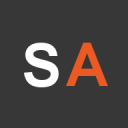
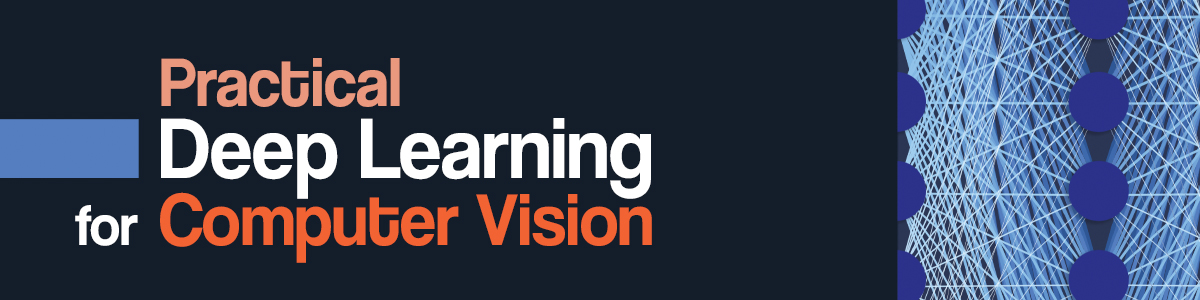
Overview
DeepDream with TensorFlow/Keras | Keypoint Detection with Detectron2 | Image Captioning with KerasNLP Transformers and ConvNets |
---|---|---|
![]() |
![]() |
![]() |
Semantic Segmentation with DeepLabV3+ in Keras | Real-Time Object Detection from Videos with YOLOv5 | Large-Scale Breast Cancer Classification |
---|---|---|
![]() |
![]() |
![]() |
When you look down at your coffee mug, you're not at all surprised by the object in front of you. You've been able to do that since you were much younger than you are now. If you can read this - you've already trained yourself in visual pattern recognition, and context recognition, stringing together words in a sentence to form a coherent message. Way before you can read, even as a baby, you can distinguish between a cat and a dog, as well as between a bird and a whale. Whether you're aware of the labels we've assigned to them ("dog", "chien", "собака", "Hund", etc.) doesn't change the fact that the features that make up a dog are different from the features that make up a whale.
Vision is one of the dominant senses in humans.
How do we imbue computers with vision? These days, deep learning is by far the most promising candidate. Computer vision is a thriving, vast and thrilling field, with discoveries being made day in and day out. The entirety of the field isn't near being solved quite yet, but the results are already superhuman.
What's Inside?
Lesson 1 - Introduction to Computer Vision
In the preface and Lesson 1 - you'll learn about the course, vision, the history of computer vision, and computer vision in general. What is computer vision and what isn't? What inspired the recent advances in computer vision? What are the general tasks, applications and tools?
Lesson 2 - Guide to Convolutional Neural Networks
In the second lesson - you'll dive into the crux of Convolutional Neural Networks (CNNs or ConvNets for short), and the theory behind them. You'll understand what convolutions are, how convolutional layers work, hyperparameters such as filter strides, padding and their effect on feature maps, pooling and fully-connected layers and how this landscape of components fits into the whole picture, as well as how these modular components can be re-used for other purposes.
Lesson 3 - Guided Project: Building Your First Convolutional Neural Network With Keras
Keras does a lot of heavy-lifting for us, and it allows us to map all of the concepts from Lesson 2 into this one in an intuitive, clean and simple way. Most resources start with pristine datasets, start at importing and finish at validation. There's much more to know.
Why was a class predicted? Where was the model wrong? What made it predict wrong? What was the model's attention on? What do the learned features look like? Can we separate them into distinct classes? How close are the features that make Class N to the feature that make Class M?
In this project, through a practical, hand-held approach, you'll learn about:
- Co-occurrence and the source of co-occurrence bias in datasets
- Finding, downloading datasets, and extracting data
- Visualizing subsets of images
- Data loading and preprocessing
- Promises and perils of Data Augmentation and Keras' ImageDataGenerator class
- Defining a custom CNN architecture
- Implementing LRFinder with Keras and finding learning rates automatically
- Evaluating a model's classification abilities
- Interpreting a model's predictions and evaluating errors
- What makes the network predict wrong
- Interpreting a model's attention maps to identify what models actually learn with tf-keras-vis and GradCam++
- Interpreting what the model's convolutional layers have learned through Principal Component Analysis and t-SNE
- How similarity search engines find similar images
Building models is largely about evaluating why they're wrong - and we'll focus the second half of the project exactly to this and evaluate our model like a pro. Here's the "concept map" of the CNN we'll train in this project, created by t-SNE:
Lesson 4 - Overfitting Is Your Friend, Not Your Foe
In this lesson, you'll learn to re-evaluate common fallacies. Most see overfitting as an inherently bad thing. It's just a thing - and the way you look at it defines whether it's "bad" or "good".
A model and architecture that has the ability to overfit, is more likely to have the ability to generalize well to new instances, if you simplify it (and/or tweak the data).
Overfitting can be used as a sanity check to check whether you have a bug in your code, as a compression method (encoding information in the weights of a neural network), as well as a general check as to whether your model can fit the data well in principle, before going further.
Lesson 5 - Image Classification with Transfer Learning - Creating Cutting Edge CNN Models
New models are being released and benchmarked against community-accepted datasets frequently, and keeping up with all of them is getting harder.
Most of these models are open source, and you can implement them yourself as well.
This means that the average enthusiast can load in and play around with the cutting edge models in their home, on very average machines, not only to gain a deeper understanding and appreciation of the craft, but also to contribute to the scientific discourse and publish their own improvements whenever they're made.
In this lesson, you'll learn how to use pre-trained, cutting edge Deep Learning models for Image Classification and repurpose them for your own specific application. This way, you're leveraging their high performance, ingenious architectures and someone else's training time - while applying these models to your own domain!
It can't be overstated how powerful Transfer Learning is, and most don't give it the spotlight it really deserves. Transfer Learning is effectively the "magic pill" that makes deep learning on small datasets much more feasible, saves you time, energy and money and a fair bit of hair-pulling. You'll learn everything you need to know about:
- Loading and adapting existing models with Keras
- Preprocessing input for pre-trained models
- Adding new classification tops to CNN bases
- The TensorFlow Datasets project and creating Train/Test/Validation splits
- Fine-Tuning Models
Lesson 6 - Guided Project: Breast Cancer Classification
With the guiding principles and concepts in hand - we can try our luck at a very real problem, that takes real lives every year. In this lesson, we'll be diving into a hands-on project, from start to finish, contemplating what the challenge is, what the reward would be for solving it. Specifically, we'll be classifying benign and malignant Invasive Ductal Carcinoma from histopathology images. If you're unfamiliar with this terminology - no need to worry, it's covered in the guided project.
We'll start out by performing Domain Research, and getting familiar with the domain we're trying to solve a problem in. We'll then proceed with Exploratory Data Analysis, and begin the standard Machine Learning Workflow. For this guide, we'll both be building a CNN from scratch, as well as use pre-defined architectures (such as the EfficientNet family, or ResNet family). Once we benchmark the most promising baseline model - we'll perform hyperparameter tuning, and evaluate the model.
You'll learn how to:
- Use
glob
and Python to work with file-system images - Work with Kaggle Datasets and download them to your local machine
- Visualize patches of images as entire slides and work with large datasets (270k images)
- How and when to handle class imbalance
- How to choose metrics, when they might be misleading and how to implement your own metrics and loss functions with Keras
- How to perform cost-sensitive learning with class weights to counter imbalance
- How to use KerasTuner to tune Keras models
In the project, we'll be building a state of the art classifier for IDC, compared to the currently published papers in renowned journals:
Lesson 7 - Guided Project: Convolutional Neural Networks - Beyond Basic Architectures
You don't need to deeply understand an architecture to use it effectively in a product.
You can drive a car without knowing whether the engine has 4 or 8 cylinders and what the placement of the valves within the engine is. However - if you want to design and appreciate an engine (computer vision model), you'll want to go a bit deeper. Even if you don't want to spend time designing architectures and want to build products instead, which is what most want to do - you'll find important information in this lesson. You'll get to learn why using outdated architectures like VGGNet will hurt your product and performance, and why you should skip them if you're building anything modern, and you'll learn which architectures you can go to for solving practical problems and what the pros and cons are for each.
If you're looking to apply computer vision to your field, using the resources from this lesson - you'll be able to find the newest models, understand how they work and by which criteria you can compare them and make a decision on which to use.
I'll take you on a bit of time travel - going from 1998 to 2022, highlighting the defining architectures developed throughout the years, what made them unique, what their drawbacks are, and implement the notable ones from scratch. There's nothing better than having some dirt on your hands when it comes to these.
You don't have to Google for architectures and their implementations - they're typically very clearly explained in the papers, and frameworks like Keras make these implementations easier than ever. The key takeaway of this lesson is to teach you how to find, read, implement and understand architectures and papers. No resource in the world will be able to keep up with all of the newest developments. I've included the newest papers here - but in a few months, new ones will pop up, and that's inevitable. Knowing where to find credible implementations, compare them to papers and tweak them can give you the competitive edge required for many computer vision products you may want to build.
By the end - you'll have comprehensive and holistic knowledge of the "common wisdom" throughout the years, why design choices were made and what their overall influence was on the field. You'll learn how to use Keras' preprocessing layers, how KerasCV makes new augmentation accessible, how to compare performance (other than just accuracy) and what to consider if you want to design your own network.
In this lesson, you'll learn about:
- Datasets for rapid testing
- Optimizers, Learning Rates and Batch Sizes
- Performance metrics
- Where to find models
- Theory and implementation of LeNet5
- Theory and implementation of AlexNet
- Theory and implementation of VGGNet
- Inception family of models
- Theory and implementation of the ResNet Family
- "Bag of Tricks for CNNs" - the linear scaling rule, learning rate warmup, ResNet variants
- Theory and implementation of Xception
- Theory and implementation of DenseNet
- MobileNet
- NASNet
- EfficientNet
- ConvNeXt
Lesson 8 - Working with KerasCV
The landscape of tools and libraries is always changing. KerasCV is the latest horizontal addition to Keras, aimed at making Computer Vision models easier, by providing new metrics, loss functions, preprocessing layers, visualization and explainability tools, etc. into native Keras objects.
The library is still under construction as of writing! Yet - it's functional, and you'll want to get used to it as soon as you can. You might even help with the development by being a part of the open source community and submit questions, suggestions and your own implementations to the project.
In this lesson - we'll be training a model with new preprocessing and augmentation layers, covering RandAug, MixUp and CutMix.
Lesson 9 - Object Detection and Segmentation - R-CNNs, RetinaNet, SSD, YOLO
Object detection is a large field in computer vision, and one of the more important applications of computer vision "in the wild". On one end, it can be used to build autonomous systems that navigate agents through environments - be it robots performing tasks or self-driving cars.
Naturally - for both of these applications, more than just computer vision is going on. Robotics is oftentimes coupled with Reinforcement Learning (training agents to act within environments), and if you want to give it tasks using natural language, NLP would be required to convert your words into meaningful representations for them to act on.
However, anomaly detection (such as defective products on a line), locating objects within images, facial detection and various other applications of object detection can be done without intersecting other fields.
Object Detection is... messy and large.
The internet is riddled with contradicting statements, confusing explanations, proprietary undocumented code and high-level explanations that don't really touch the heart of things. Object detection isn't as straightforward as image classification. Some of the difficulties include:
- Various approaches: There are many approaches to perform object detection, while image classification boils down to a similar approach in pretty much all cases.
- Real-time performance: Performing object detection should oftentimes be in real-time. While not all applications require real-time performance, many do, and this makes it even harder. While networks like MobileNet are fast, they suffer in accuracy.
- Devices: The speed-accuracy tradeoff is extremely important for object detection, and you might not be able to know on which devices it'll run on, as well as the processing units that will be running them.
- Libraries and implementations: Object detection is less standardized than image classification, so you'll have a harder time finding compatible implementations that you can just "plug and play".
- Formats: Labels for classification are simple - typically a number. For detection - will you be using COCO JSON, YOLO-format, Pascal VOC-format, TensorFlow Detection CSV, TFRecords? Some are in XML, while others are in JSON, Text, CSV or proprietary formats.
- Utilities: With classification - you just spit out a number. With detection - you're typically expected to draw a bounding box, plot the label and make it look at least somewhat pretty. This is more difficult than it sounds.
In this lesson, you'll learn:
- Two-stage object detection architectures such as R-CNN, Fast R-CNN and Faster R-CNN
- One-stage object detection architectures such as SSD, YOLO and RetinaNet
- The difference between SSD and YOLO
- Several examples of object detection, instance segmentation, keypoint detection or overviews of PyTorch's
torchvision
, Ultralytic's YOLOv5, TFDetection, Detectron2, Matterport's Mask R-CNN - Object detection metrics
Lesson 10 - Guided Project: Real-Time Road Sign Detection with YOLOv5
Building on the knowledge from the previous lesson - we'll make use of the most popular and widely used project in the industry, and utilize YOLOv5 to build a real-time road sign detection model, that works on videos. In this project, you'll learn about:
- Ultralytic's YOLOv5, sizes, configurations, export options, freezing and transfer learning
- Training on Roboflow Datasets and using Roboflow
- Data Collection, Labelling and Preprocessing
- Tips on labelling data for object detection
- Training a YOLOv5 detector on custom data
- Deploying a YOLOv5 Model to Flask and preparing for deployment for Android and iOS
Lesson 11 - Guided Project: Image Captioning with CNNs and Transformers
In 1974, Ray Kurzweil's company developed the "Kurzweil Reading Machine" - an omni-font OCR machine used to read text out loud. This machine was meant for the blind, who couldn't read visually, but who could now enjoy entire books being read to them without laborious conversion to braille. It opened doors that were closed for many for a long time. Though, what about images?
While giving a diagnosis from X-ray images, doctors also typically document findings such as:
"The lungs are clear. The heart and pulmonary are normal. Mediastinal contours are normal. Pleural spaces are clear. No acute cardiopulmonary disease."
Websites that catalog images and offer search capabilities can benefit from extracting captions of images and comparing their similarity to the search query. Virtual assistants could parse images as additional input to understand a user's intentions before providing an answer.
In a sense - Image Captioning can be used to explain vision models and their findings.
So, how do we frame image captioning? Most consider it an example of generative deep learning, because we're teaching a network to generate descriptions. However, I like to look at it as an instance of neural machine translation - we're translating the visual features of an image into words. Through translation, we're generating a new representation of that image, rather than just generating new meaning. Viewing it as translation, and only by extension generation, scopes the task in a different light, and makes it a bit more intuitive.
Framing the problem as one of translation makes it easier to figure out which architecture we'll want to use. Encoder-only Transformers are great at understanding text (sentiment analysis, classification, etc.) because Encoders encode meaningful representations. Decoder-only models are great for generation (such as GPT-3), since decoders are able to infer meaningful representations into another sequence with the same meaning. Translation is typically done by an encoder-decoder architecture, where encoders encode a meaningful representation of a sentence (or image, in our case) and decoders learn to turn this sequence into another meaningful representation that's more interpretable for us (such as a sentence).
In this guided project - you'll learn how to build an image captioning model, which accepts an image as input and produces a textual caption as the output.
Throughout the process, you'll learn about:
-
KerasNLP - a Natural Language Processing parallel of KerasCV
-
The Transformer architecture, as compared to RNNs, token and position embedding, and using Transformer encoders and decoders
-
Perplexity and BLEU Scoring
-
Preprocessing and cleaning text
-
Vectorizing text input easily
-
Working with the
tf.data
API to build performantDataset
s -
Building Transformers from scratch with TensorFlow/Keras and KerasNLP - the official horizontal addition to Keras for building state-of-the-art NLP models
-
Building an image captioning hybrid model
Lesson 12 - Guided Project: DeepLabV3+ Semantic Segmentation with Keras
Semantic segmentation is the process of segmenting an image into classes - effectively, performing pixel-level classification. Color edges don't necessarily have to be the boundaries of an object, and pixel-level classification only works when you take the surrounding pixels and their context into consideration.
In this Guided Project, you'll learn how to build an end-to-end image segmentation model, based on the DeepLabV3+ architecture and cover:
- Semantic segmentation architectures
- Implement U-Net with Keras
- Atrous convolutions and the Atrous Spatial Pyramid Pooling Module
- The Albumentations library and augmenting semantic segmentation maps with images
- Creating semantic segmentation datasets with masks as labels
- Implementation of the DeepLabV3+ architecture
- Segmentation metrics and loss functions
Lesson 13 - DeepDream - Neural Networks That Hallucinate?
Hierarchical abstraction appears to be what our brains do, with increasing support in the field of Neuroscience. While some protest drawing lines between the computation the brain does and silicone computing found in computers, some support the parallels, such as Dana H. Ballard in his book "Brain Computation as Hierarchical Abstraction", who works as a Computer Science professor at the University of Texas, with ties to Psychology, Neuroscience and the Center for Perceptual Systems.
Inspired by hierarchical abstraction of the visual cortex, CNNs are hierarchical, and hierarchical abstraction is what allows them to do what they do. Exploiting exactly this property is what allows us to create a really fun (and practical) algorithm, and the focus of this lesson. It's called DeepDream, because we associate odd, almost-there-but-not-there visuals with dreams, and the images are induced by a deep convolutional neural network.
In this lesson, you'll learn about the DeepDream algorithm, with gaussian gradient smoothing, and learn to create images such as:
Yet Another Computer Vision Course?
We won't be doing classification of MNIST digits or MNIST fashion. They served their part a long time ago. Too many learning resources are focusing on basic datasets and basic architectures before letting advanced black-box architectures shoulder the burden of performance.
We want to focus on demystification, practicality, understanding, intuition and real projects. Want to learn how you can make a difference? We'll take you on a ride from the way our brains process images to writing a research-grade deep learning classifier for breast cancer to deep learning networks that "hallucinate", teaching you the principles and theory through practical work, equipping you with the know-how and tools to become an expert at applying deep learning to solve computer vision.
How the Course is Structured
The course is structured through Guides and Guided Projects.
Guides serve as an introduction to a topic, such as the following introduction and guide to Convolutional Neural Networks, and assume no prior knowledge in the narrow field.
Guided Projects are self-contained and serve to bridge the gap between the cleanly formatted theory and practice and put you knee-deep into the burning problems and questions in the field. With Guided Projects, we presume only the knowledge of the narrower field that you could gain from following the lessons in the course. You can also enroll into Guided Projects as individual mini-courses, though, you gain access to all relevant Guided Projects by enrolling into this course.
Once we've finished reviewing how they're built, we'll assess why we'd want to build them. Theory is theory and practice is practice. Any theory will necessarily be a bit behind the curve - it takes time to produce resources like books and courses, and it's not easy to "just update them".
Guided Projects are our attempt at making our courses stay relevant through the years of research and advancement. Theory doesn't change as fast. The application of that theory does.
In the first lessons, we'll jump into Convolutional Neural Networks - how they work, what they're made of and how to build them, followed by an overview of some of the modern architectures. This is quickly followed by a real project with imperfect data, a lesson on critical thinking, important techniques and further projects.
Lessons
Downloadable Resources
Course Ebook (PDF)(60 MB) | Enroll to download | ||
Lesson 2 Notebook(942 KB) | Enroll to download | ||
Lesson 3 Notebook(21 MB) | Enroll to download | ||
Lesson 4 Notebook(1 MB) | Enroll to download | ||
Lesson 5 Notebook(2 MB) | Enroll to download | ||
Lesson 6 Notebook(3 MB) | Enroll to download | ||
Lesson 7 Notebook(11 MB) | Enroll to download | ||
Lesson 8 Notebook(2 MB) | Enroll to download | ||
Lesson 9 Notebook(4 MB) | Enroll to download | ||
Lesson 10 Notebook(2 MB) | Enroll to download | ||
Lesson 11 Notebook(1 MB) | Enroll to download | ||
Lesson 12 Notebook(3 MB) | Enroll to download | ||
Lesson 13 Notebook(30 MB) | Enroll to download | ||
Lesson 14 Notebook - Unoptimized(73 KB) | Enroll to download | ||
Lesson 14 Notebook - Optimized(312 KB) | Enroll to download |