Going Further - Resource Recommendations
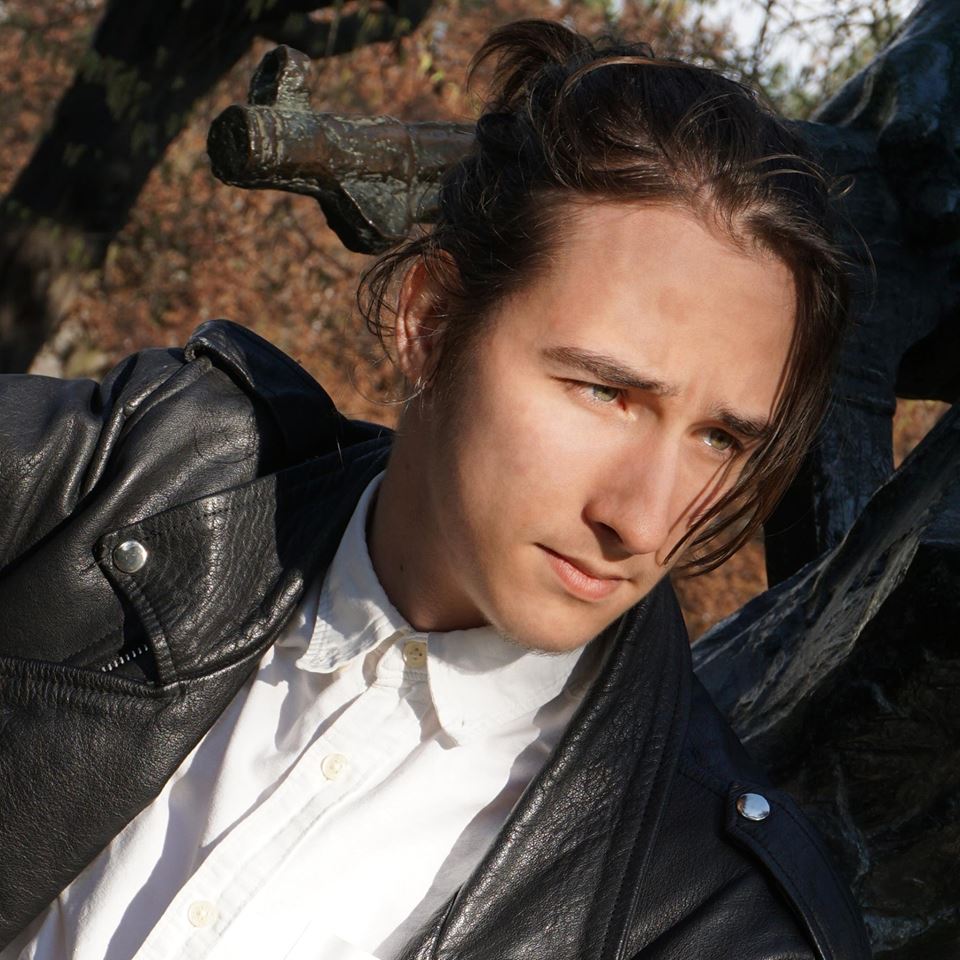
We've gone far from the first chapter! From the foundations of computer vision, how it can be conceptualized, to building your first CNN classifier, validating and testing it, through several projects, discovering landscapes, building highly performant data and augmentation pipelines, intersecting NLP and CV, reading and implementing papers, applying best practices, etc.
Deep Learning is a large field, and you might want to dive deeper into it, or you might be looking to dive deeper into Machine Learning in general, or Data Science as the parent field to it. This course is intended for a varied audience, as mentioned in the beginning, and with that assumption - I've made choices on what to include and what not to include, to maximize the informational value for everyone involved.
One of the assumptions was that you have at least a basic understanding of ML and DL and that there's no need to cover material such as activation and loss functions, what a neural network is, etc. Condensing it all into a small section at the start of the course wouldn't do it justice, nor would it really help people get a grasp on it, so I've omitted it. This is the section in which I'd love to take a moment to point you to a few resources that could help you deepen your grasp on data science in general, as well as other resources on deep learning for computer vision.