Building a Transformer-Based, CNN-Powered Image Captioning Model
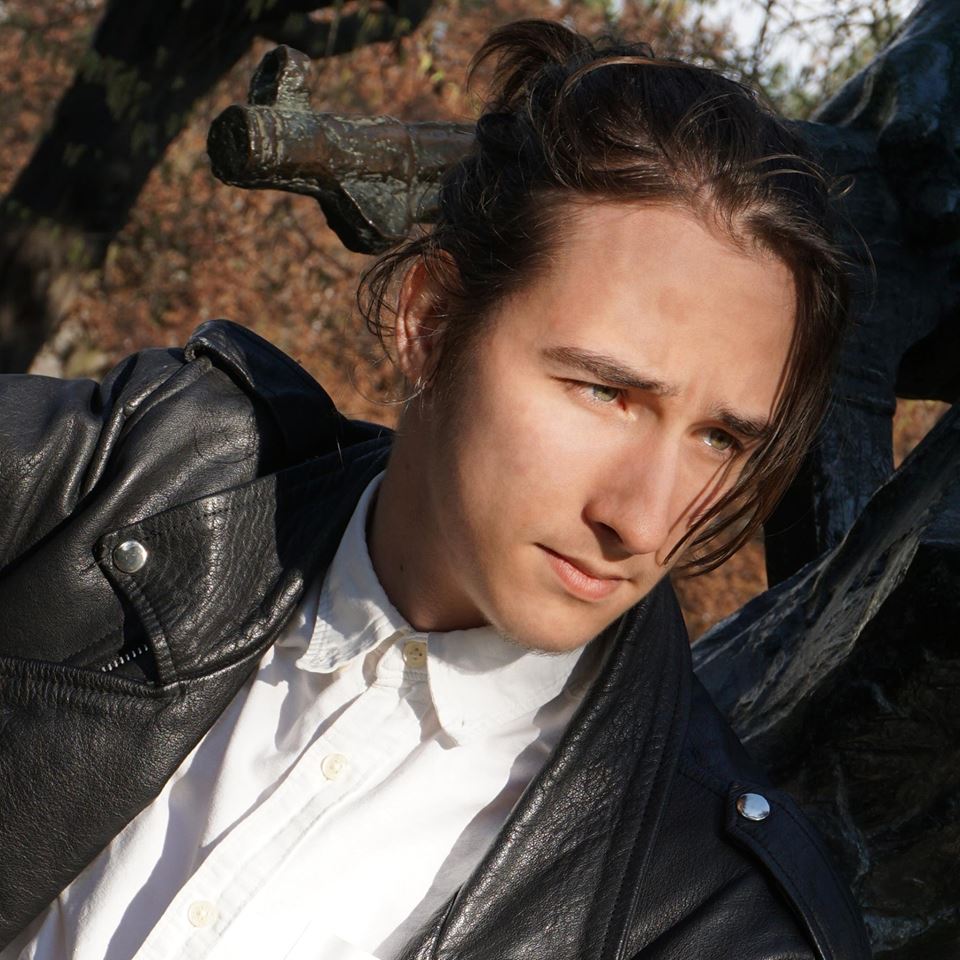
David Landup
With our datasets primed and ready to go - we can define the model. Using KerasNLP, we can fairly easily implement a transformer from scratch:
# Encoder
encoder_inputs = keras.Input(shape=(None,))
x = keras_nlp.layers.TokenAndPositionEmbedding(...)(encoder_inputs)
encoder_outputs = keras_nlp.layers.TransformerEncoder(...)(inputs=x)
encoder = keras.Model(encoder_inputs, encoder_outputs)
# Decoder
decoder_inputs = keras.Input(shape=(None,))
encoded_seq_inputs = keras.Input(shape=(None, EMBED_DIM))
x = keras_nlp.layers.TokenAndPositionEmbedding(...)(decoder_inputs)
x = keras_nlp.layers.TransformerDecoder(...)(decoder_sequence=x, encoder_sequence=encoded_seq_inputs)
x = keras.layers.Dropout(0.5)(x)
decoder_outputs = keras.layers.Dense(...)(x)
decoder = keras.Model([decoder_inputs,encoded_seq_inputs], decoder_outputs)
# The output of the transformer is the output of the decoder
transformer_outputs = decoder([decoder_inputs, encoder_outputs])
transformer = keras.Model([encoder_inputs, decoder_inputs], transformer_outputs)
The input is followed by TokenAndPositionEmbedding()
and either a TransformerEncoder
or TransformerDecoder
. The model is put together by feeding the output of the encoder into the decoder, besides the input it already gets, which is embedded positionally. This architecture is pretty much a perfect reflection of the diagram from the paper. Now, we'll have to make a few tweaks here - transformers work on sequences, and our images aren't sequences, nor are the feature maps output by a ConvNet.
Start project to continue